
BRONCO EMBER
Bronco Ember is a NASA TechLeap Autonomous Observation Challenge Winner.
​
Bronco Ember is a nascent wildfire detection system that uses a combination of artificial intelligence, edge computing, infrared imaging, and a 2 degree of freedom gimbal. The system is designed to be small and compact while still being able to provide large impact to the wild fire prevention and detection industry.The system has already undergone its first test flight that provided successful results and a proving ground to continue development on the emerging technology. The system is udergoing continued development to refine and improve the system that is capable of identify a fire that is less than 20 feet in diameter in 5 minutes or less after first ignition.
MEET THE LEADS



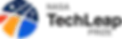
CHALLENGES FACED
​
One of the significant challenge we faced was obtaining imagery of consistent quality across various remote sensing platforms, resulting in variations in ground sampling distance (GSD) within the training dataset for the Al model's computer vision training set. Consistency in GSD is crucial for machine learning training data sets that can benefit from similarly sized pixel groups, improving the accuracy and performance of the Al model. However, due to limitations and variations in remote sensing platforms, acquiring imagery with similar events imaged(wildfires) and GSD proved to be a significant hurdle in our project. Despite this challenge, we persevered by carefully addressing and mitigating the impact of variable GSD within the training dataset, striving to optimize the Al model's performance in accurately identifying wildlife presence.
​
Throughout our project, we remained determined to overcome the time-consuming image transfer and manual masking process, as well as the variations in GSD. We recognized the significance of reliable and accurately labeled training data for training the Al model effectively. By navigating these challenges and implementing measures to mitigate their impact, we aimed to enhance the performance of our Al model and improve its ability to identify and analyze wildlife signs accurately.
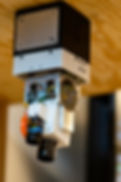
**Finalized Ember Model